DuckDB: the Rise of In-Process Analytics and Data Singularity
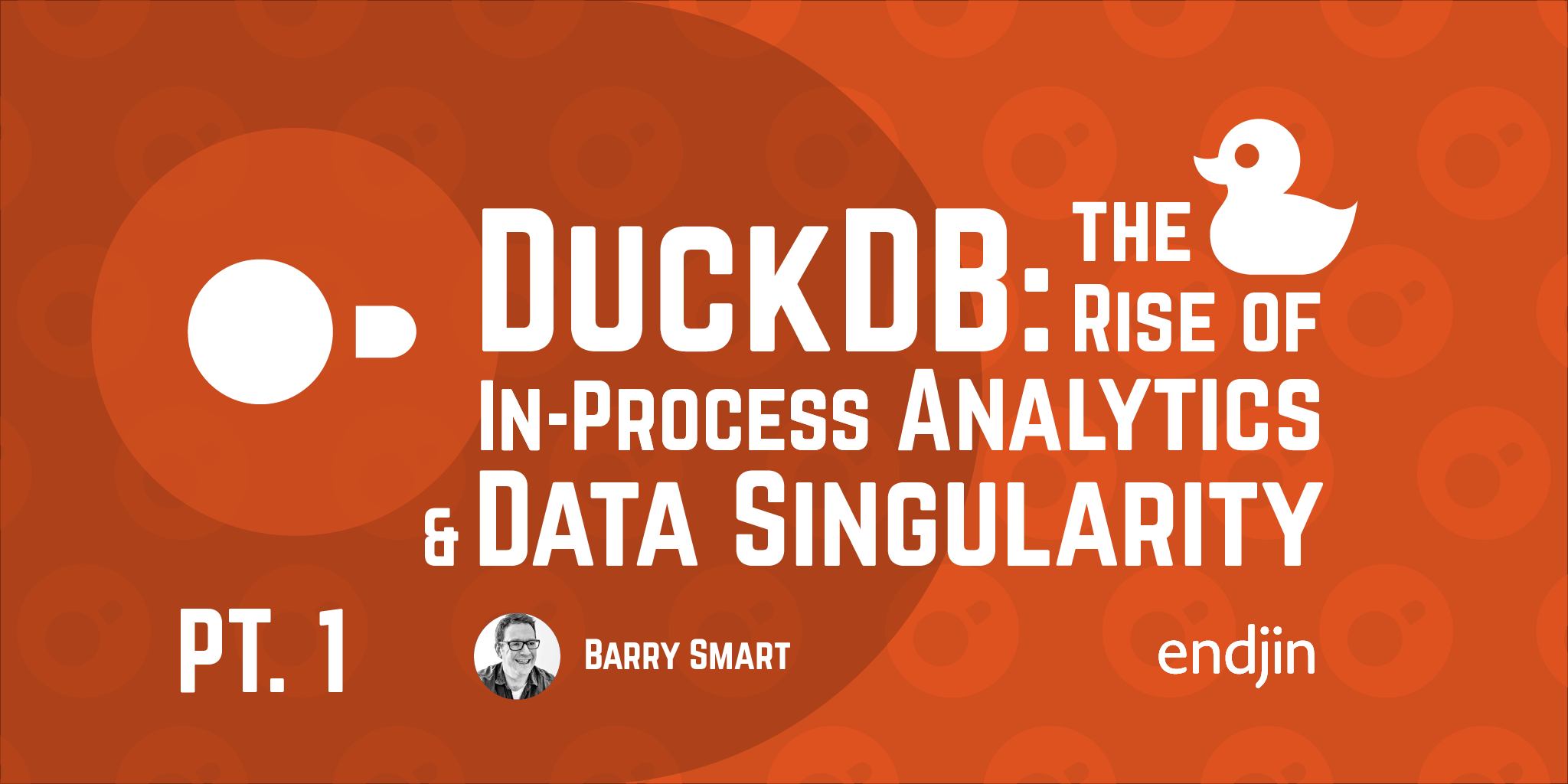
TL;DR:
We're witnessing a fundamental shift in data processing where modern hardware capabilities are outpacing the growth of analytical datasets for many use cases. This "data singularity" means single-node solutions can now handle workloads that previously required distributed systems. DuckDB exemplifies this trend as an in-process analytical database that runs directly within your application, offering SQL power with exceptional performance for datasets up to ~1B rows, challenging the way we approach architecting, developing and deploying analytics solutions.
The Fundamental Shift
For decades, conventional wisdom in data analytics suggested that serious data work required dedicated database servers. Need to analyze a large dataset? Export it, set up a database connection, load the data, deal with type conversions, and finally run your analysis. This workflow introduced significant friction — what Hannes Mühleisen, co-creator of DuckDB, co-founder and CEO of DuckDB Labs and Professor of Data Engineering at the University of Nijmegen, aptly describes as "slower and more frustrating" than necessary.
The rise of Big Data platforms like Hadoop and Apache Spark further entrenched this mindset. These distributed processing frameworks were revolutionary, but they came with significant overheads. Only companies with deep pockets could afford the capital outlay for infrastructure and the specialized DevOps teams to set up and maintain a complex array of infrastructure.
The latest generation platforms such as Databricks and Microsoft Fabric are lowering barrier to Big Data engines such as Apache Spark and modern lakehouse architectures through PaaS and more recently SaaS offerings, with pay as you go commercial models. This has lowered the barrier to entry significantly.
However, it can often feel like the compute engine provided by these platforms is overkill for "small to medium sized" workloads. These Big Data platforms we use were originally designed to process data volumes that today would still be considered to be truly Big Data. For example, Hadoop was designed to process Web Server Log files which 1 Terabyte each: Yahoo had 25,000 servers to process 25 Petabytes of data, so using these platform can often feel like a sledgehammer to crack a nut for most SME workloads.
The trend of working with "smaller data" is also being driven by modern analytics architectures such as Data Mesh, where we are being encouraged to move away from centralised monolithic data warehouses (or lakehouses) towards more granular data products. To support this more focused approach to delivering analytics, we want tools that can work at "data lake scale" but allow us to work in an agile fashion. For example to:
- Load only the data we need from the Bronze area of the lake;
- Transform it through the Silver and Gold layers of the lake;
- Project an actionable insight that allows a specific audience to achieve a specific goal.
Furthermore, as software engineers, we crave a local development experience. This is technically possible with Apache Spark (which can be installed locally through Python packages), but it becomes unwieldy when you want to apply good engineering practices such as implementing automated unit and integration tests.
There's also growing concern about the cost, both financial and environmental, of using heavyweight distributed compute engines. Spinning up a cluster of servers to process a few million rows of data raises questions about total cost of ownership and carbon footprint, especially when modern hardware might be capable of handling the same workload on a single node.
This has led to a new generation of in-process analytics tools — including DuckDB for SQL enthusiasts and libraries like Polars for DataFrame aficionados — that are designed to maximize the capabilities of modern hardware. These tools represent different approaches to the same challenge: how can we process larger datasets efficiently without the overhead of distributed systems?
This brings us to a provocative question: What if there's another way? What if the processing power of our everyday machines has outpaced the growth of common analytical datasets?
The Moore's Law Observation
Mühleisen presents a compelling argument: the computing capabilities of our laptops and workstations have been growing exponentially (following Moore's Law), while the size of most analytical datasets we work with daily grows at a much slower pace. Modern laptops come equipped with multiple CPU cores, substantial RAM, and blazing-fast SSD storage — resources that often remain underutilized when using traditional client-server data platforms.
Consider these specifications of my amazing Surface Laptop Studio 2:
- 20 CPU cores
- 64GB RAM
- NVMe SSDs capable of 5+ GB/s read speeds
With such computational firepower at our fingertips, do we really need to offload processing to external servers for many common analytical tasks?
Mühleisen proposes a fascinating concept: we are approaching a "data singularity" where the processing power of mainstream single-node machines such as laptops will surpass the requirements of the vast majority of analytical workloads.
His thought experiment: If the entire working population of the Netherlands (about 10 million people) typed data continuously for a year, they would produce roughly 200 terabytes of text — about 60 terabytes when compressed. This would fit on just two modern hard drives, and likely on a single laptop's storage within a decade.
The growth in "useful" human-generated data is fundamentally limited by human capacity, while computing power continues to grow exponentially. The implication is clear: for most analytical workloads, the future may not be distributed big data platforms, but incredibly efficient single-node processing.
This is supported by real-world evidence. Amazon's internal data, analysed in a recent "The Redshift Files: The Hunt For Big Data" blog post, suggests that the vast majority of analytical datasets are well within the processing capabilities of modern single-node machines when using optimized software. Perhaps more concerning is the observation that:
We're spending 94% of query dollars on computation that doesn't need big data compute.
Enter DuckDB: Analytics Without the Friction
DuckDB represents a fundamental rethinking of how analytical databases should work — a new generation of data processing tools that brings analytical power directly to where you're working. Instead of operating as a separate server process, DuckDB runs directly within your application process — whether that's Python, R, Java, or others. This remarkable versatility extends even further: thanks to its small footprint, DuckDB can run in your web browser via WebAssembly, on mobile devices, and has even been embedded in automotive software. This flexibility opens exciting opportunities for analytics "at the edge" where data is generated.
Another killer feature is that it is cloud native, offering the ability to read data directly from remote cloud storage infrastructure such as Amazon S3, Azure Data Lake Storage or Fabric OneLake. Allowing you to treat data on your enterprise data lake as if the files were hosted locally - we'll focus on this specifically in our next blog.
In the examples in this blog, we have embedded DuckDB in a Jupyter notebook through a few simple steps:
pip install duckdb
Then:
import duckdb
It's as simple as that, a real game changer! The more you use it, the more benefits you start to discover. This "in-process" architecture eliminates network overhead, authentication complexities, and the entire client-server communication layer.
Unlike the previous generation of Big Data tools that were designed to scale out across clusters, DuckDB is designed to scale up and make the most of modern hardware capabilities. It represents a refreshing counter-trend in a field that had become fixated on distributed computing, even when it wasn't always necessary.
The Story Behind the Name
There's a charming story behind DuckDB's name. Hannes Mühleisen, the co-founder, lives with his family on a boat in Amsterdam. When he wanted a pet that would be compatible with boat life, he chose a duck named Wilbur. Although Wilbur eventually grew up and moved on, the database was named in his honour. This personal touch reflects the human-centered approach that permeates the entire project.
DuckDB has quickly become one of the fastest-growing database systems of all time, used by companies of all shapes and sizes across diverse industries.
The Culture Behind the Code
What makes DuckDB special isn't just its technical prowess, but the culture that drives its development. With its roots in academia (originating at CWI, the Dutch national research center where Python was invented), the DuckDB team brings a unique approach to database development:
- Academic Rigor: The team publishes research papers on their innovations, grounding their work in computer science principles
- Engineering Excellence: Highly optimized C++ code and vectorized processing reflect a commitment to performance
- Community Focus: The team is deliberately friendly and constructive in their interactions, avoiding the "grumpy" culture that can plague some open source projects
- Leading by Example: Despite being a small team of only about 20 people, they've created an outsized impact through focus and efficiency
This culture of excellence combined with approachability has helped build a vibrant community around the project. It's a testament to what a small, focused team can achieve when they prioritize both technical excellence and a positive user experience - a great example of a "small team achieving big things"!
Open Source with a Sustainable Model
Open Source Software is at the core of DuckDB. It is released under the MIT license, making it freely available for any use. This bucks the trends of other database products which have the technical prowess and the academic foundation, but remain closed source (Cosmos DB is one example, whilst there is an open source variant it's not the original multi-modal version, but a v-Core variant).
Rather than taking the venture capital route, DuckDB Labs (the company which now employs the creators of DuckDB) has focused on sustainable growth through support and consulting services.
They've also established the DuckDB Foundation as a non-profit to ensure the project's long-term viability and protect its open-source nature. This foundation owns the intellectual property, ensuring that DuckDB remains open source.
This model allows users to build on DuckDB with confidence in its long-term availability, without vendor lock-in concerns.
It remains to be seen if this model can be sustained, we can see plenty of companies (such as MotherDuck) profiting directly from DuckDB. We hope that they, and others in the growing community benefiting from DuckDB, will contribute to funding it over the long term to give it longevity and allow it to continue to thrive.
The Growing Ecosystem
DuckDB has recently opened up its extension system to community contributions, creating an ecosystem of specialized tools that extend its capabilities. These extensions can add:
- New file formats and protocols
- Specialized functions
- Additional SQL syntax
- Custom compression algorithms
- Integrations for AI workloads such as vector based indexing and search
This extensibility will further cement DuckDB's position as a central processing engine that connects various data tools and formats.
This ecosystem extends beyond DuckDB to the wider data and analytics community. For example, you can orchestrate DuckDB transformations using DBT, Microsoft Fabric Python Notebook runtime comes pre-installed with DuckDB and Ontop (the virtual knowledge graph) can be used to bridge from tabular data in DuckDB to a knowledge graph.
Key Benefits of DuckDB's Approach
Simplicity: Install with a single command (
pip install duckdb
) with zero dependencies or configuration. Meaning you can host it anywhere you can run Python (other languages that are supported) including in your notebooks!User-Friendliness: Designed with a focus on being approachable and intuitive, DuckDB prioritizes developer experience with helpful error messages, sensible defaults, and a forgiving syntax that makes it accessible to both SQL experts and newcomers.
Cloud native: a growing suite of extensions enable you to connect seamlessly with cloud storage, cloud databases and other cloud services.
Batteries Included: a fully-featured database management system with transactions, persistence, and a comprehensive set of SQL operators.
Performance: by running within your application process and leveraging vectorized execution across multiple CPU cores, DuckDB achieves remarkable speed—often outperforming heavyweight distributed systems for datasets up to hundreds of gigabytes.
Transparent Resource Management: can gracefully use disk space when data doesn't fit in memory, without requiring user intervention.
Integration: direct, zero-copy access to Pandas and Polars DataFrames in Python means you can query these structures without costly data transfers.
Format Support: native handling of CSV, Parquet, JSON files with intelligent type inference takes the friction out of loading data from common raw data sources.
Portability: A single file format makes sharing analytical databases as simple as "emailing a file". Whilst this is not something we would encourage, it reinforces the point that when a DuckDB is persisted to the file system, you have a highly transportable, self-contained collection of artefacts: curated data stored in tables, well defined data types and other constraints such as relationships, bundled with value adding views layered on top.
Standards-based: Full SQL support with advanced features like window functions, pivoting, and more.
As Mühleisen puts it, DuckDB "just works" — it's like magic to many users because it removes so much friction from the analytical workflow.
Finding DuckDB's Sweet Spot
DuckDB fills a crucial gap in the data processing spectrum:
- Spreadsheets (like Excel): great for small datasets up to ~100K rows;
- Traditional Python packages (like pandas): handle medium datasets up to ~10M rows;
- DuckDB: comfortably processes large datasets up to ~1B rows on a single machine;
- Distributed systems (like Apache Spark): required for truly massive datasets beyond single-node capabilities.
This middle range, anything up to a few Billion rows, is where DuckDB truly shines. This middle range actually spans 99% of the data sets we work with, and it's a space where, until recently, data practitioners were forced to use heavyweight distributed systems that introduced significant complexity and overhead, despite the fact that the dataset could theoretically fit on a single powerful machine.
DuckDB excels at solving practical, everyday challenges that create friction for data professionals - in a nutshell it's suited to both the ETL and analytical workloads:
- Loading raw data from files;
- Converting between file formats (CSV to Parquet);
- Filtering large datasets before further processing;
- Performing complex operations such as joins;
- Running exploratory data analysis on multi-gigabyte files;
- Acting as the analytics engine in a data intensive application.
These seemingly simple operations can be surprisingly cumbersome with traditional tools. For instance, writing a single Parquet file from Apache Spark requires understanding distributed execution concepts, shuffle operations, and partition management—complexity that's unnecessary for many use cases.
As Mühleisen notes, these "small simple use cases" come up time and again, and DuckDB removes the barriers to just getting work done.
What's Coming Next
In this first part of our three-part series on DuckDB, we've explored the concept of the data singularity and introduced DuckDB as a tool that embodies this shift toward powerful single-node analytics. We've looked at the cultural and philosophical foundations of the project and identified where it fits in the data processing ecosystem.
In the next article, we'll dive deeper into the technical details of DuckDB, exploring its columnar architecture, vectorized execution, SQL enhancements, and the performance optimizations that make it exceptionally fast. We'll look at benchmarks comparing it to distributed systems and explain the specific features that make it stand out for analytical workloads.
The third and final part will focus on practical applications, showing how to integrate DuckDB with enterprise environments including Microsoft Fabric, exploring architectural patterns it enables, and providing concrete examples of how it can reduce total cost of ownership in real-world scenarios.