DuckDB in Depth: How It Works and What Makes It Fast
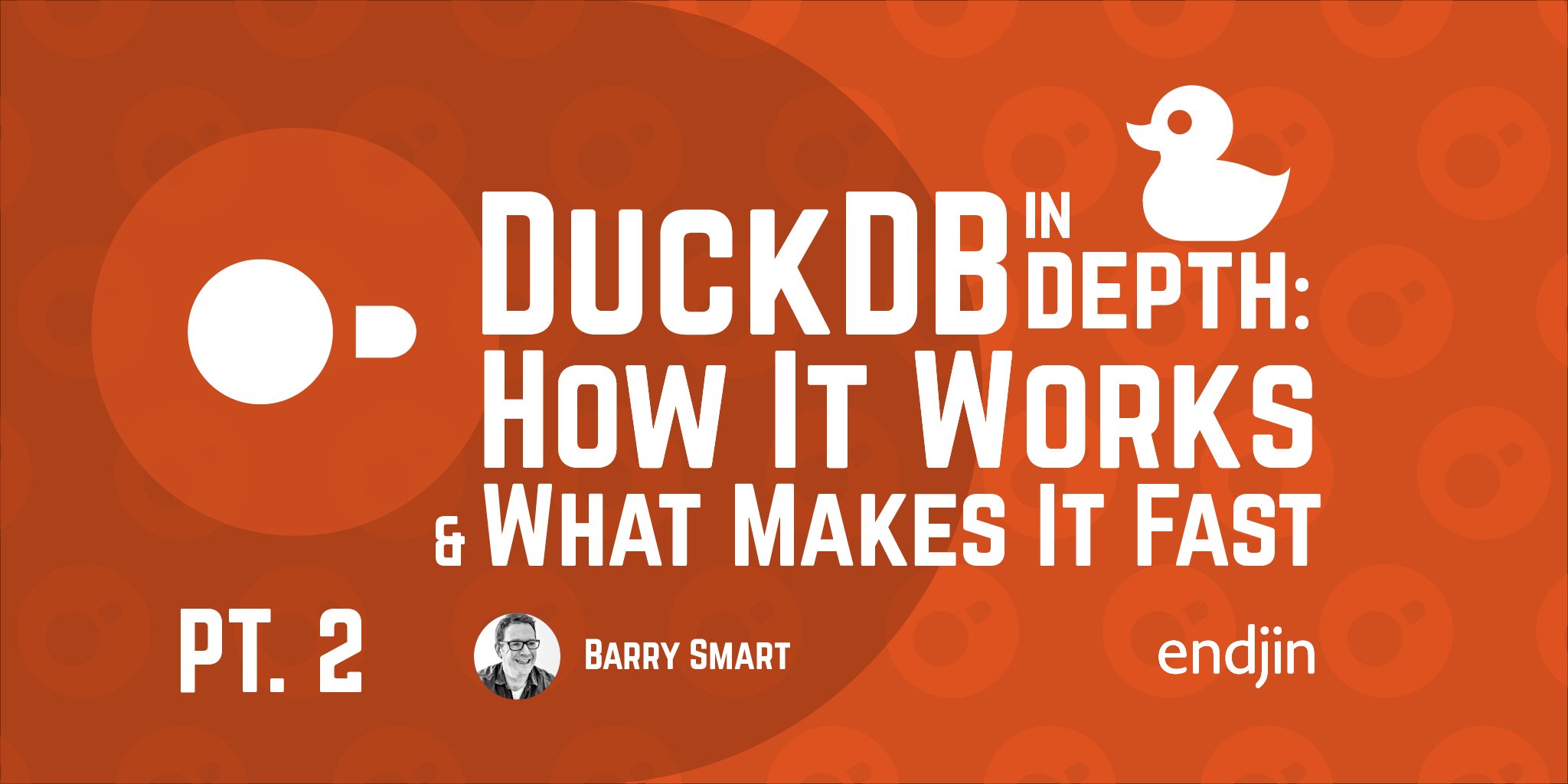
TL;DR:
DuckDB achieves remarkable performance through a purpose-built C++ engine with columnar storage architecture and vectorized execution optimized for modern CPUs. It intelligently uses all available cores, employs zone maps to skip irrelevant data, and manages I/O efficiently for larger-than-memory workloads. These optimizations, combined with quality-of-life SQL enhancements and advanced database features, enable DuckDB to outperform distributed systems for many analytical tasks while maintaining a developer-friendly experience that reduces friction in the data workflow.
A Quick Recap: The Data Singularity
In Part 1 of this series, we introduced the concept of the "data singularity" – the point where the processing power of single-node machines surpasses the requirements of most analytical workloads. We explored how DuckDB, an in-process analytical database, exemplifies this trend by leveraging modern hardware capabilities to process datasets up to a billion rows directly within your application process.
Now it's time to look under the hood and understand what makes DuckDB so exceptionally fast and user-friendly. The performance of DuckDB isn't magic – it's the result of deliberate engineering choices and modern optimization techniques built on decades of database research.
The Columnar Advantage
Under the hood, DuckDB employs a columnar storage architecture - a critical design choice for analytical workloads. Unlike traditional row-based databases (SQLite, PostgreSQL), DuckDB stores each column separately, enabling:
- Better compression (low cardinality data can be compressed highly efficiently)
- Column pruning (read only the columns you need)
- Vectorized operations optimized for modern CPUs
This design aligns perfectly with analytical queries that typically scan large portions of data but only need a subset of columns.
Smart Data Loading
One of DuckDB's most impressive features is its multi-hypothesis CSV parsing. This technology allows DuckDB to intelligently parse most files without human intervention — correctly inferring types, handling edge cases, and doing it all with remarkable speed.
For data scientists and analysts who spend significant time just getting data into a usable state, this feature alone can save hours of frustration. The summarize
command further enhances this workflow, quickly generating comprehensive statistics across all columns in large datasets.
Purpose-Built C++ Engine
Unlike many data tools that are built atop existing libraries, DuckDB was written from scratch in C++ 11 with no external dependencies. This disciplined approach allowed the team to:
- Maintain complete control over performance-critical code paths
- Ensure consistent behavior across all platforms
- Achieve exceptional portability without compromise
The codebase adheres to strict design principles, with required dependencies (like regex handling) carefully "vendored in" to the project rather than relying on external libraries that could vary across platforms.
Vectorized Execution for Cache Efficiency
DuckDB employs vectorized execution, a technique made possible on modern compute hardware that processes data in small batches (typically 1024-2048 items) rather than row-by-row or entire-column-at-once:
- These vector sizes are carefully chosen to fit within CPU L1 cache (32-128KB per core on modern processors)
- Operations work on vectors of data, maximizing CPU instruction throughput
- The codebase is written to enable auto-vectorization by modern compilers, which translate the code into SIMD (Single Instruction, Multiple Data) operations
This approach avoids both the inefficiency of row-by-row processing and the memory pressure of processing entire columns at once, striking an optimal balance.
Parallel Processing and Thread Management
DuckDB automatically parallelizes operations across all available CPU cores:
- Queries are broken down into tasks that can run concurrently
- Data is processed in "row groups" that are distributed across threads
- Even a simple operation like reading a CSV file will utilize all available cores
- This parallelization happens transparently without user configuration
Zone Maps for Selective Scanning
One of DuckDB's clever optimizations is the creation of "zone maps" (also known as min-max indexes):
- For each column in each row group, DuckDB tracks minimum and maximum values
- When filtering data (e.g.,
WHERE date > '2023-01-01'
), entire row groups can be skipped if their min/max values show there can't be any matches - This is particularly effective for ordered or semi-ordered columns like dates, IDs, or timestamps
- It dramatically reduces I/O and processing for many common queries
Intelligent I/O Management
DuckDB gracefully handles datasets larger than available RAM:
- It streams data from storage rather than requiring everything to fit in memory
- When intermediate results need to be materialized, it can transparently spill to disk
- It uses memory-mapped files for efficient access to on-disk data
- For cloud storage, it employs optimized access patterns like HTTP range requests to fetch only the data it needs
Together, these optimizations allow DuckDB to push the boundaries of what's possible on a single machine, often matching or exceeding the performance of distributed systems for many real-world analytics tasks.
SQL Enhancements: Removing Friction
DuckDB doesn't just implement standard SQL, it thoughtfully extends it with quality-of-life improvements that make everyday tasks more intuitive. These small but impactful enhancements save time and reduce cognitive load. They reflect DuckDB's fixation on optimizing the developer experience, making database interactions more intuitive and removing unnecessary friction. Rather than forcing users to adapt to the database, DuckDB adapts to how people naturally want to work with their data.
In all of the examples below, for brevity, we have left out the Python wrapper you would place around the SQL, for example the full Python snippet to execute the first example query would be:
duckdb.sql("SELECT * FROM file_name.csv")
Simplified File Access
In the following example, DuckDB checks if a table with the name file_name.csv
exists. If not, it looks for file with that name and infers from the suffix that it is most likely a CSV file. This straightforward syntax automatically reads the CSV file, unleashing DuckDB's "CSV sniffer" which takes much of the trickiness out of loading data from CSV.
SELECT * FROM file_name.csv
Or even simpler:
FROM file_name.csv
This is a shortcut for SELECT * FROM file_name.csv
, further streamlining exploratory data analysis.
It is just as simple to load other file types such as loading JSON where you have options to fully decompose the elements of a record like JSON structure into a tabular structure or load less structured JSON data into a single column.
Again there are is a lot of intelligence built into the read_json
method which automatically determines the best way to load the data and will also assign appropriate types:
SELECT * FROM read_json('my_file.json')
DuckBD can also load multiple files in one operation through support for the glob syntax or by providing a list of files to read.
In many enterprise cases, you may have an upstream process fire-hosing data onto your lake in parquet format. Here is an example of how you could use DuckDB to query those through use of globbing to load multiple files in a single operation:
SELECT * FROM read_parquet('source=SAP/entity=invoices/year=*/*.parquet');
We're really only scratching the surface of all of the file loading features that DuckDB provides above. But hopefully the examples are enough to illustrate how much effort has been put into removing the friction from loading data from common file formats and common use cases.
Smarter Column Selection
When working with wide tables, it's common to want all columns except a few. Traditional SQL requires you to explicitly list every column you want to keep:
SELECT column_a, column_b, column_c, column_d, column_e, column_g, ...
FROM table_name
DuckDB offers a much more elegant solution with the EXCEPT syntax:
SELECT * EXCEPT (column_f, column_r)
FROM table_name
This selects all columns except the ones specified in parentheses, making your queries more succinct and easier to maintain. If the table structure changes (new columns added), your query will automatically include those new columns without modification.
Smarter Grouping
The GROUP BY ALL
syntax automatically groups by all non-aggregated columns in the SELECT clause (in this case, country and region), eliminating redundant typing and reducing errors:
SELECT country, region, SUM(sales)
FROM sales_data
GROUP BY ALL
Beyond Basic Processing: The Database Features You Actually Want
DuckDB brings many advanced database features to the analytical workflow without the traditional database overhead:
Data Integrity and Relationships
One significant advantage of DuckDB over dataframe libraries is its ability to formalize relationships and constraints in your data. It brings key data integrity concepts from traditional OLTP database technology into the analytical space:
- Foreign Key Constraints: Define and enforce relationships between tables
- Primary Keys: Ensure unique identification of rows
- Check Constraints: Validate data against predefined rules
- Not Null Constraints: Ensure required fields contain values
These constraints help maintain data quality in analytical workflows, something that's often missing in pure dataframe-based approaches, making them behave in unpredictable ways when poor data quality data is allowed into a pipeline.
There's much more to table technology than just running queries over single tables, and DuckDB provides these powerful features without the complexity of a full database server.
Replacement Scan: Seamless DataFrame Integration
One of DuckDB's most valuable attributes is how it elegantly bridges the SQL and DataFrame worlds. In today's polyglot data engineering environments, teams often find themselves working across multiple paradigms such as SQL and DataFrame libraries like pandas or Polars. DuckDB offers a unifying solution that respects both paradigms, though it particularly shines for those with SQL expertise.
An example of this is DuckDB's "replacement scan" feature represents a particularly elegant solution for bridging SQL and DataFrame worlds. When you reference a table in a SQL query that doesn't exist in the database, DuckDB intelligently looks for DataFrames in the host environment with that name:
import duckdb
import pandas as pd
# Create a DataFrame, in this case using Pandas
my_df = pd.DataFrame({'a': [1, 2, 3], 'b': [4, 5, 6]})
# Query it directly with SQL - no explicit conversion needed
result = duckdb.sql("SELECT * FROM my_df WHERE a > 1")
What makes this special is that:
- It works without copying data ("zero-copy" access), making it extremely efficient
- It's completely transparent to the user
- It allows SQL experts to work with DataFrames using familiar syntax
- It enables a natural workflow where you can switch between dataframe and SQL at will
This seamless integration serves as a practical bridge between SQL-centric and DataFrame-centric ways of working, allowing each team member to use the paradigm they're most comfortable with.
For SQL experts, DuckDB provides all the power and expressiveness of SQL with thoughtful extensions that make it even more productive. For DataFrame users, the seamless integration with Pandas and Polars allows them to move between tabular processing paradigms without friction. This versatility makes DuckDB an excellent choice for small multi-disciplinary data teams.
Portable Database Format
The file format in which DuckDB stores its databases (including tables, views, and constraints) is extremely portable. This enables convenient workflows like:
- Emailing a small analytical database to a colleague
- Distributing analytical assets as a single file
- Backing up entire datasets with a simple file copy
This portability addresses a common pain point in analytics where sharing both data and its structure often requires complex export/import procedures and supporting documentation (to explain relationships or constraints).
Parquet Integration
Converting between formats is trivial: COPY my_file.csv TO my_file.parquet
. Parquet offers significant advantages over CSV:
- Columnar storage (10-100x smaller file sizes)
- Schema enforcement
- Much faster read times
- Better handling of complex data types
Making DuckDB am attractive option for Bronze to Silver transformations in a medallion architecture where a key part of that process is to standardise the data.
Views and Macros
DuckDB allows you to create views (virtual tables defined by queries) and macros (reusable SQL snippets) that travel with your database file. This means you can encapsulate complex logic once and share it with colleagues without them needing to understand all the underlying complexity.
Transactions
Full support for SQL transactions brings reliability to analytical workflows. Made a mistake in your data transformation? Simply roll back. This feature, traditionally associated with OLTP systems, proves remarkably useful for iterative analytical work.
Understanding DuckDB's Limitations
While DuckDB offers impressive capabilities, it's important to understand its design constraints and limitations:
Single-Writer Concurrency Model
DuckDB supports transactions, but its concurrency model allows only one writer at a time:
- Multiple readers can access a database simultaneously
- Only one process can write to a database at any given moment
- Attempting multiple concurrent writers will result in an error - this is a deliberate design choice that simplifies many aspects of the system
For analytical workflows where batch processing is common, this is rarely a significant limitation. However, it does mean DuckDB isn't suitable for high-concurrency transactional workloads with many simultaneous writers - which is not a use case that it was designed to support!
Single-Node Architecture
DuckDB is designed as a single-node solution:
- It doesn't distribute work across multiple machines
- While it scales up effectively (utilizing more cores/memory on one machine), it doesn't scale out
- For truly massive datasets (hundreds of terabytes or petabytes), distributed systems remain necessary
This limitation is intentional: by focusing exclusively on single-node performance, DuckDB can avoid the complexity and overhead of distributed coordination.
Memory and Resource Management
While DuckDB can handle datasets larger than available RAM, there are practical considerations:
- Performance will degrade when working with datasets significantly larger than memory because swapping from memory to disk kills performance
- Very complex operations on very large datasets may still exhaust system resources
- The user is responsible for ensuring the host machine has sufficient disk space for temporary files
Not a Replacement for All Database Needs
DuckDB is not designed to replace:
- High-concurrency OLTP databases (like PostgreSQL or MySQL)
- Multi-petabyte data warehouses (like Fabric Lakehouse, Snowflake or BigQuery)
- Real-time streaming processors (like Kafka or Flink)
Understanding these limitations helps position DuckDB appropriately in your data architecture—as a powerful tool for specific analytical needs rather than a universal database solution.
Read Only From Delta
At the time of writing this blog, DuckDB only supports reading from Delta.
However, there is a means of writing to Delta by first converting a DataFrame library such as Polars and then writing that to Delta:
import duckdb
import polars as pl
from deltalake import DeltaTable, write_deltalake
df = duckdb.query("SELECT * FROM read_csv('my_local_file.csv');").pl()
polars_dataframe.write_delta(f"./my_delta_table", df)
Performance Reality Check
Claims about performance deserve scrutiny, but benchmarks consistently show DuckDB delivering impressive results. In a comparison with Apache Spark (a distributed processing framework) on the TPC-H benchmark:
- DuckDB on a single machine completed the benchmark in 1 minute 16 seconds
- Spark on a cluster of 32 machines took around 8 minutes
Even more telling: when scaling Spark to 1,024 cores across many machines, it still couldn't match DuckDB's single-machine performance due to the coordination overhead of distributed computing.
This performance gap exists because distributed systems introduce unavoidable costs:
- Network communication between nodes
- Coordination and synchronization overhead
- Data serialization and deserialization
- Task scheduling and management
For many analytical workloads, these distributed computing costs outweigh the benefits — a reality that DuckDB's designers recognized and addressed by focusing on maximizing single-node efficiency.
What's Coming Next
In this second part of our three-part series on DuckDB, we've explored the technical details that make DuckDB so fast and user-friendly. We've looked at its columnar architecture, vectorized execution, parallel processing capabilities, and the thoughtful SQL enhancements that reduce friction in analytical workflows.
In the final article of this series, we'll focus on practical applications of DuckDB, exploring how to integrate it with enterprise environments including Microsoft Fabric. We'll examine architectural patterns it enables, how it can serve as a building block in larger solutions, and how it bridges between local development and enterprise deployment. Stay tuned for concrete examples of how DuckDB can reduce total cost of ownership and fit into modern data architectures.
Whether you're a SQL enthusiast looking for more performant tools or a data team looking to streamline your processes, the next article will provide practical guidance on making the most of DuckDB in real-world scenarios.