Data and AI Engineering Maturity - Fix our problems before we hit the buffers
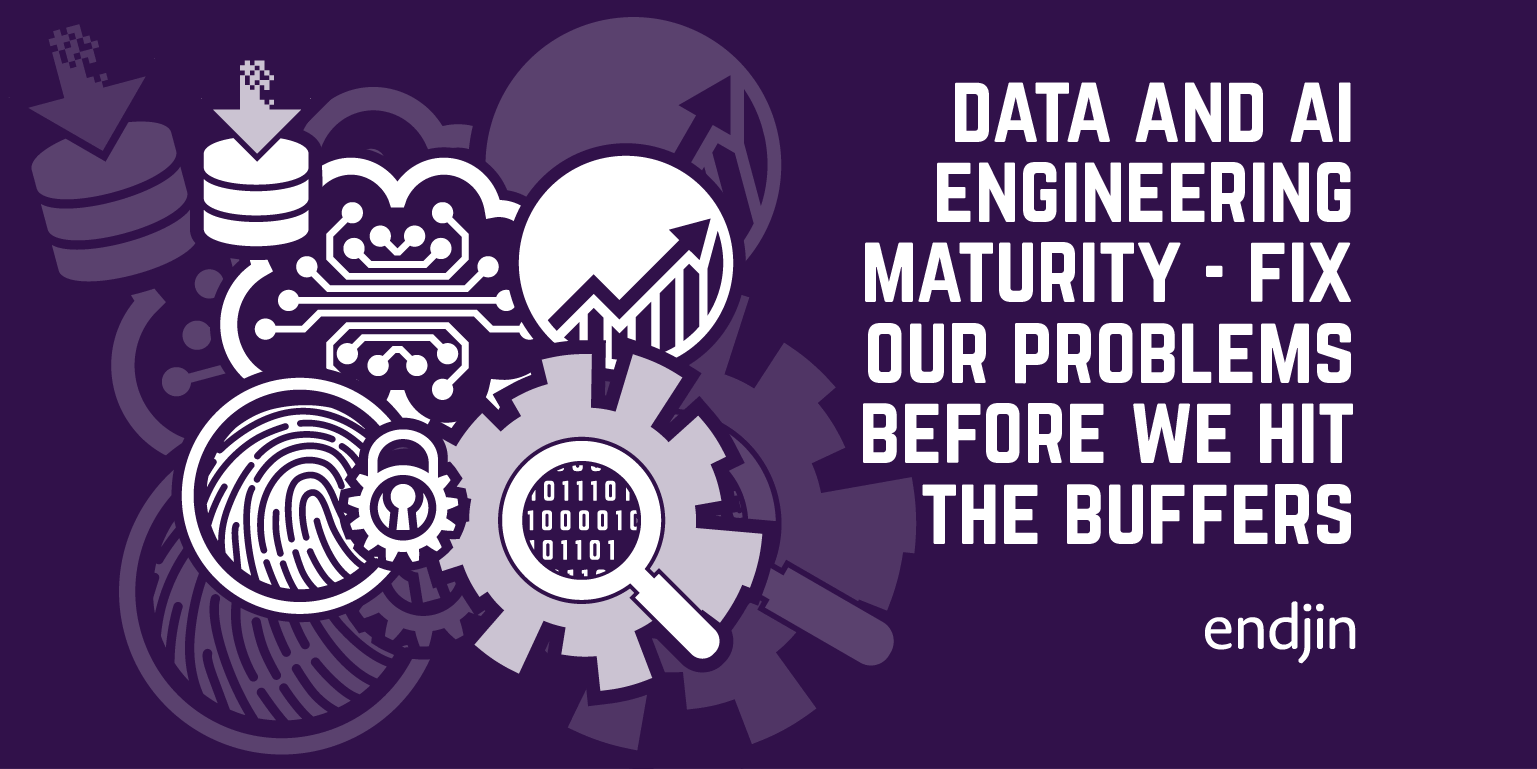
Something has happened over the last 10 years: business has started to view "data" as the engine of change.
In the 1980s and 1990s "information technology" and "the micro on every desktop" held that position. Over 10-15 years "the IT department" evolved methodologies for providing and managing those services more efficiently (with varying degrees of success!).
Today, we have automated, virtualized infrastructure (often provided by mega-vendors in "the Cloud"). Instead of provisioning hardware, we have Bring-Your-Own-Device policies (from laptops to smartphones). Working-from-home has taken facilities out of the corporate headquarters and into the community. All of this has turned IT into a commoditized service on which the business relies in much the same way as it does electricity. The specialists that make it work are hidden from sight, and often not even employed by the consuming organization.
Through the 2000s, the business focus shifted from "IT" to "software". Gradually, every business became a software business. So, software became the engine of change.
This meant that the software engineering capabilities of "ordinary" businesses suddenly became the throttle (or the brake!) accelerating growth and productivity. Even as IT was still optimizing, the software engineering lifecycle became a business-critical problem. The industry looked to manufacturing and process engineering bringing concepts like Kanban, Agile, and Six Sigma to the mainstream.
Of course, IT and Software Engineering aren't "solved problems" - far from it. Understanding business needs and picking appropriate methods are as challenging as ever - but we have well-evolved methodological tools to help us do the job (e.g. Wardley Doctrine).
In that context, we are now seeing another shift; and "data" (especially when it is wearing dark glasses and a trilby and going by the name of "AI") is viewed by businesses as the new engine of change.
However, it is fair to say, the world of data is very much in the early stages of the maturity cycle. The industry is still very "technology" focused. Appropriate processes, controls and methods are in their infancy.
The socio-technical aspects are poorly understood. Practitioners have been very much "back room technologists" (and developed deep skills in that domain) but they are now being asked to step closer to the business and understand complex end-user needs. "Democratization" vastly increases the expected pace of change, and the complexity of governance and control.
To avoid crashing into the trough of disillusionment (a real and present risk given the current hype-cycle), we need to mature rapidly. Senior data technologists need to learn to speak the language of business, and develop the methods that help the business to understand the risk and opportunities in data-driven transformation (particularly managing the non-binary nature of success and failure in a statistical domain). They need to find their allies in finance and operations, and learn how to develop ubiquitous language to share understanding.
Having attended a number of conference talks, and read extensively in the blog-o-sphere (in part thanks to Ed's excellent Power BI Weekly - you should subscribe if you haven't already), people have clearly got the right idea. Concepts that have been developed in the software domain are starting to be talked about in the data space. But, while we are hearing many of the right words, the core ideas are often confused and misapplied - just as they were at the start of the journey in software engineering.
This is no criticism of the practitioners in the data space - it is great to see that the journey is underway.
But it is a warning; if you are retreating into your technology comfort zone, or see "a tool" as your saviour in any area in which you may feel weaker today - then you should challenge your thinking.
When you hear an "expert" talking about a concept new to the domain (such as 'Swiss Cheese Security' or 'Top-Down/Bottom-Up Governance') - don't take their interpretation as "correct". Go back to the original sources and read the relevant papers. Draw your own conclusions. Deeply understand the concepts. Discuss them as widely as possible. Challenge assumptions - especially if the conclusions being proposed make the status quo feel "comfortable".
In particular, in our capacity as (senior) technologists and change managers, we need to avoid pushing responsibility for understanding the needs onto the business. It is our job to lift ourselves out of the technical domain, and translate its constraints and possibilities, risk and value, into the language used by our colleagues in the rest of the organization.
The nuances are complex, and our job is to bring clarity and shared understanding.
The opportunities to avoid repeating the mistakes of the past, in other domains, are great. But we will fail if we don't challenge ourselves at these early stages.