Copilot - Are You Ready to Unleash the Power of AI in Self Service Analytics?
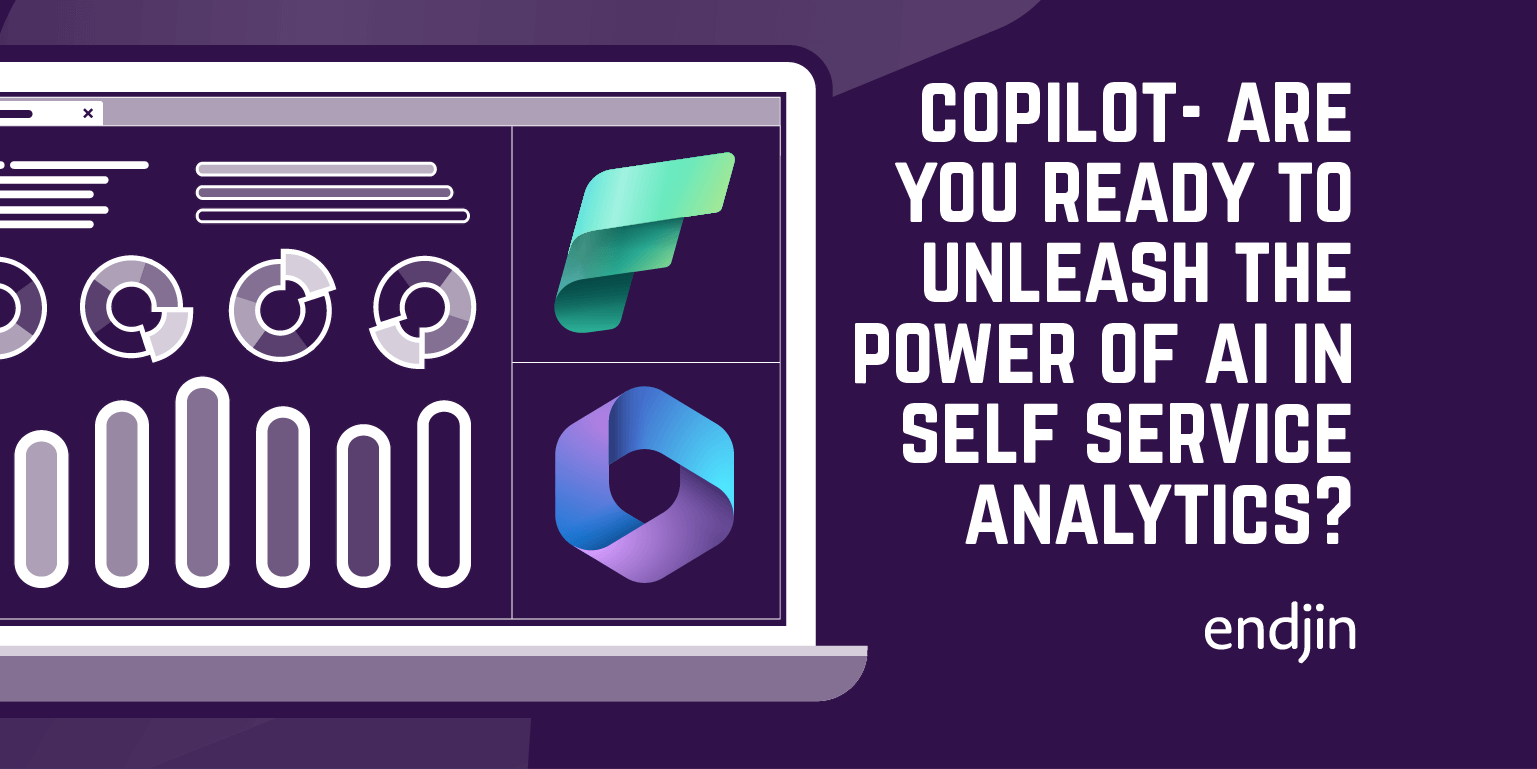
Copilot in Power BI and Microsoft Fabric is impressive. Of course, it's still very early and your mileage may vary between the polished marketing demos and your real world experience. But, history has shown us that this is only going to improve - just look at GitHub Copilot - over time, with usage, training and incremental improvements it will turn into something genuinely useful and closer to the nirvana vision of the demos.
You could think of this new AI-powered capability as the next generation of self service analytics. This is something that "power users" (or what we might nowadays call citizen analysts) have always wanted. This means the ability to ingest, visualise, and slice and dice data in ways that work for them. Not bound by pre-canned reports, or a dependency on data engineering or the wider enterprise, but flexibility to interrogate and analyse any data in any way to surface new insights.
The game changing step is that Copilot will allow you do this via natural language prompts, such as:
"Help me build a report summarising our sales growth rate, quarter on quarter for the last 2 years"
"What was the gross profit margin in EMEA in Q1 this year?"
"What are the biggest drivers for growth across our subscription-based products?"
So, if you can now ask for anything you want, and get it immediately, does this mean you no longer need to be bound knowing upfront the exact data sets, dimensions, attributes or data aggregations that you care about to feed into your reporting? Or, even more significantly, can you bypass your data engineers/data teams entirely?
The promise of self service analytics has always been a tough one. At the enterprise level, anyone who's been involved in any sizeable data project initiative will know that designing a perfect data model to serve all needs - any type of query, at any scale - isn't really possible. The design process (whether that's database design or data modelling more generally) needs to be driven by a set of constraints. At a high level, these are the classic fundamental elements of project management - time, cost and scope (or quality, depending on your preferred flavour). The scope one is most interesting here, as it implies that we need to know what the consumers of our data platform/data models want to do with them.
Fulfilling the requirement of being able to slice your data by any dimension is clearly a big ask (much more so that than dealing with a sub-set of core dimensions for targeted reporting) so you can't avoid the hard questions around understanding what people really want to do. But it's not just functional scope that will have an impact. Optimising a data model for performant querying largely depends on the type of queries you're going to execute - time-series querying might need a very different underlying data structure to aggregation-by-category and the impact of those choices is amplified as the size of your data increases.
If we're talking about ad-hoc analytics - the "power user" flow that's increasingly supported by no-code/low-code tooling, a lot of that formality goes out the window - there naturally isn't going to be a lot of standardisation going into reports that are quickly put together to meet a transient business need. And without standardisation, automation becomes more difficult, which will no doubt impact the usefulness of any AI-driven output.
But a big consideration in all cases is that natural language processing requires a well understood ubiquitous domain language in your model - the semantic layer that is being queried needs to reflect a common view on how we see, understand and use the data, otherwise we won't get the results that we're expecting.
Another dimension to this is that AI based, language driven processes will require business users / citizen data analysts to develop prompt engineering skills in order to get the outputs they want, rather than just verbalising internal mental models. When you add that even seemingly well understood industry-wide definitions can vary wildly from organisation to organisation (we once worked with a team that had 10 different definitions of gross margin), at both ends of the process, the most important thing is making sure that everyone is talking the same language. And if you belong to an organisation where people don't actually speak the same language, this becomes significantly harder once you factor in cultural and semantic nuances.
The point I'm trying to make is that unleashing new levels of capability and power at the citizen analyst level is great. But, it doesn't take away the need for trying to understand what it is that they're trying to achieve (and why), the need for solid governance foundations, properly designed data models (where appropriate) and a common language to underpin it all - or to put it another way, the "socio" part of the "sociotechnical" aspect of modern data-driven organisations.
In many ways, the increased agility (and productivity that comes with it) makes it even more important to get all of this right. When done well, this could unlock incredible new value across your organisations. But if not, the impact could be just as significant in a negative way - data quality issues exaggerated, trust in data eroded, and confidence in the data teams and initiatives being lost. At best, technology being seen as gimmicky leading to missed opportunities, but at worst, potentially damaging to your business.
So - how do we achieve the nirvana and avoid the chaos? Well, nothing's really changed on that front - whilst the technology is evolving to make it easier to surface insights, the hard work isn't in the technology - it's still all about people.
If you're in the "enterprise reporting" space, endjin's Insight Discovery process is critical - it's designed to help you ask the right questions of the business so that your data projects are set up for success. It deals with the starting point that is accepted all too often - that the business users of the system can't tell you exactly what they want. By putting the consumer and the outputs front and centre of your data initiatives, you'll design data products that deliver real value, and can be supercharged with AI.
More generally, a well-established Centre of Enablement team that can advocate and evangelise about good internal reporting practices and align on common terminology and language across the business will introduce standardisation in the ad-hoc analytics space. And remember, the challenges also come with opportunities - if Copilot can reduce the burden on the scarce resources of an internal data team, this gives you more capacity to focus on the 5 V's (volume, value, variety, velocity, and veracity) of your data, which will absolutely help with the above.
Ultimately, the future of self service analytics lies in finding the right balance between human expertise and AI-driven capabilities. By embracing this balance, organizations can realise the benefits of a new era of data-driven decision-making, unlocking transformative insights while ensuring data integrity, reliability, and user satisfaction.